April 2023

Algorithms are increasingly being used in social media platforms for various purposes such as recommendations and amplifying movements, but they can also be used as vectors of harm. The misuse of generative AI to create deepfakes, voice clones, and synthetic media can lead to misleading content, and algorithmic overdependence can create filter bubbles and echo chambers, affecting marginalized communities. Governments are taking measures to mitigate these harms through regulations, such as the EU AI Act, Digital Services Act, and legislation in the US. Lawsuits against social media platforms for algorithmic harms are also being seen, potentially setting a precedent for holding them liable. The article emphasizes the need for trustworthy AI systems that are developed with ethics and harm mitigation in mind.
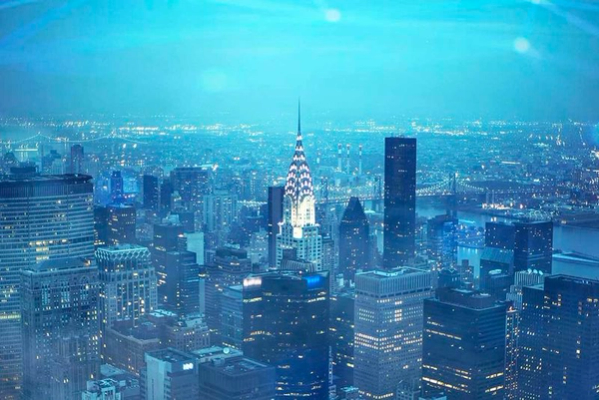
New York City has enacted Local Law 144, regulating automated employment decision tools (AEDTs) used to evaluate applicants or employees. The law requires yearly bias audits to assess the tool's disparate impact on marginalized groups. Employers and employment agencies must also comply with notification requirements and provide notice of the use of the tool. Penalties for non-compliance start at $500 for the first violation. New Jersey has proposed a similar bill, and the New York State Assembly has also introduced legislation requiring annual bias audits. Holistic AI recommends taking steps early to ensure compliance ahead of the laws coming into effect.
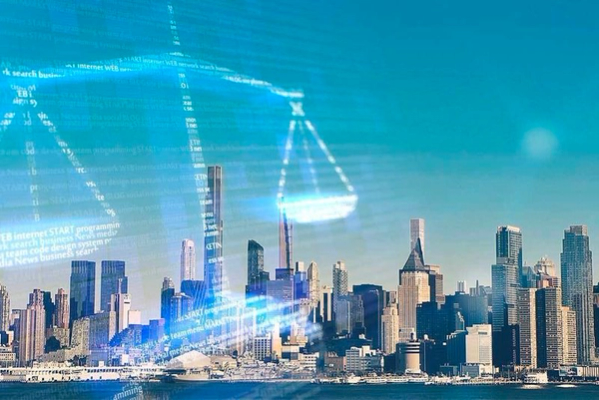
The New York City Department of Consumer and Worker Protection will enforce its final rules on the Bias Audit Law beginning on July 5, 2023. These rules clarify definitions, modify the calculation of scores, and establish new regulations for independent auditors. The definition for "machine learning, statistical modelling, data analytics, or artificial intelligence" has been expanded, and the requirement for inputs and parameters to be refined through cross-validation or training and testing data has been removed. The adopted rules also require auditors to indicate missing data and exclude categories that comprise less than 2% of the data while justifying the exclusion. The summary of results must also include the number of applicants in each category. Historical data may only be utilized if the employer provides it to the auditor or if the AEDT has never been used before, while test data may only be used if no historical data is available.
March 2023
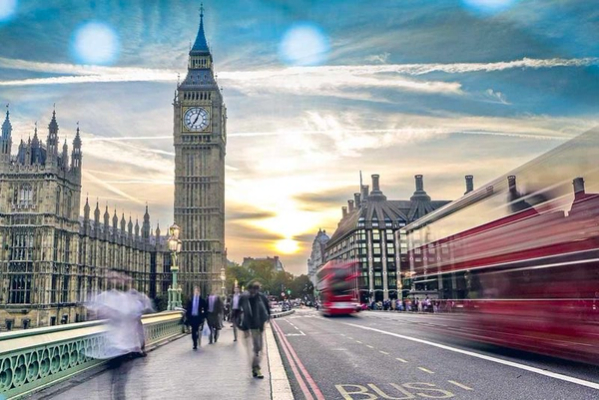
The UK Government has published a White Paper outlining a regulatory framework for AI, based on five key principles of safety, transparency, fairness, accountability and contestability. The approach seeks to promote responsible innovation and maintain public trust. The White Paper establishes a multi-regulator sandbox and recommends practical guidance to help businesses put these principles into practice.
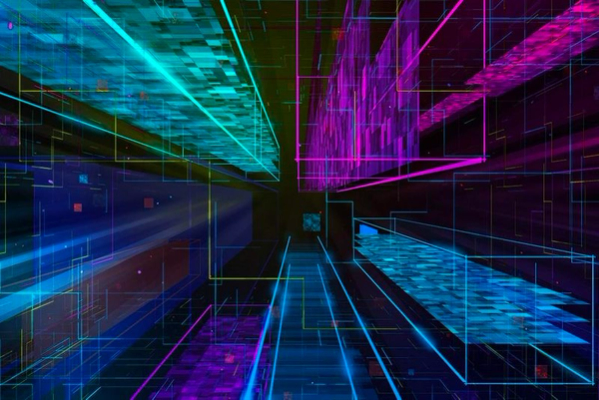
In critical areas such as healthcare and self-driving cars where AI is being increasingly used, the efficacy of algorithms is crucial. The measurements of efficacy depend on the type of system and its output. Classification systems rely on metrics such as true and false positives and negatives, accuracy, precision, recall, F1 scores, and area under the operating curve. For regression systems, correlations and root mean square error are used to compare outputs with ground truth scores. The choice of metric depends on the context and type of model being used. Holistic AI's open-source library provides built-in metrics for measuring model performance.