December 2022
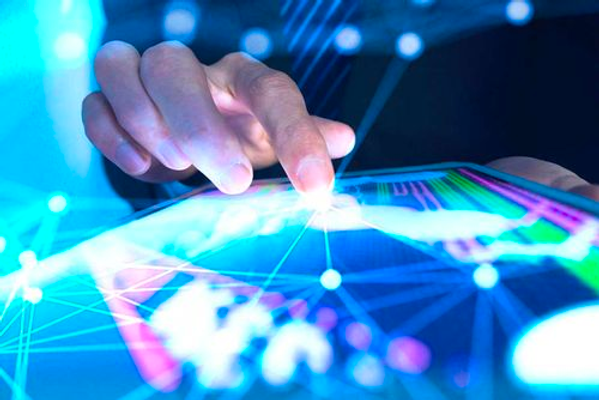
21 Dec 2022
The article discusses the current regulatory environment surrounding artificial intelligence (AI) and the need for AI auditing to ensure the safety, legality and ethics of AI systems. The process of AI auditing involves four stages, including triage, assessment, mitigation, and assurance. The assessment phase evaluates the efficacy, robustness and safety, bias, explainability, and algorithm privacy of the system. The audit outcomes are used to inform the residual risk of the system, and mitigation actions are suggested to address the identified risks. The importance of conducting an audit of an AI system is highlighted, including improving stakeholder confidence and trust and future-proofing systems against regulatory changes.
August 2022
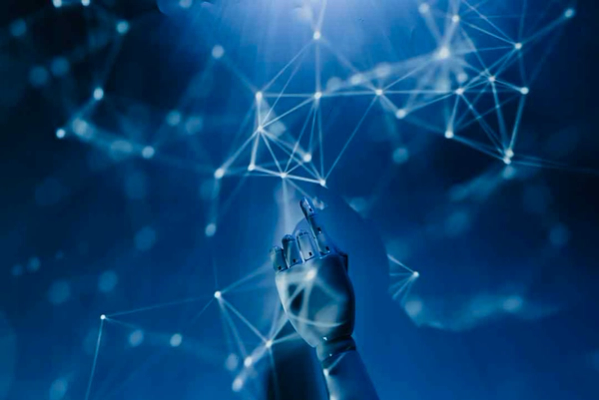
The use of algorithms and automation brings about many benefits, but it also poses risks, as shown in high-profile cases of harm associated with their use. Applying AI ethics principles could have prevented these harms from occurring. Cases that highlight the risks associated with algorithms include the COMPAS tool, Amazon's scrapped resume screening tool, and Apple's algorithm used to determine credit limits. AI ethics principles could have been useful in mitigating these issues if bias assessments and checks for differential accuracy for subgroups were conducted. It stresses the importance of transparency and explainability when it comes to automated decision tools and the assurance of algorithms to reduce the harm that can result from their use.
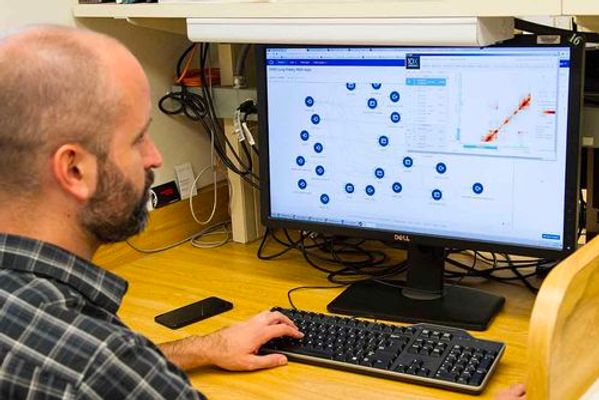
Impact assessments, including Algorithmic Impact Assessments (AIAs) and Data Protection Impact Assessments (DPIAs), can be used to determine the potential risks and harm associated with a system. AIAs are used to identify the risks associated with the use of an automated decision system, while DPIAs are used to assess data management strategies. These assessments are increasingly being used to determine the impact of AI systems on users and stakeholders. EU AI Act requires AIAs to determine significant risks posed by high-risk systems to fundamental rights, health, or safety. A higher impact system is subject to tighter regulations and stricter requirements, and low impact systems are subject to more lenient requirements.
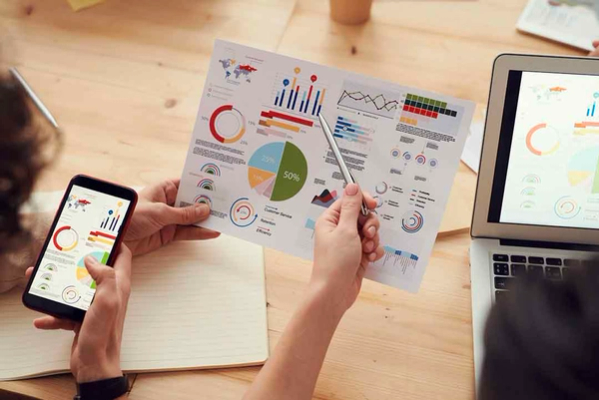
The rise in AI use has led to concerns about its legal, ethical, and safety implications, resulting in the emergence of AI ethics. Algorithm auditing is an approach to mitigating potential harm that can arise from high-risk AI systems such as those in healthcare, recruitment, and housing. Auditing involves assessing an algorithm's safety, ethics, and legality and consists of five stages of development: data and set up, feature pre-processing, model selection, post-processing and reporting, and production and deployment. Following the audit, assurance is the process of determining whether the AI system conforms to regulatory, governance, and ethical standards, including certification, impact assessments, and insurance. Whilst auditing contributes to assuring an algorithmic system, governance and impact assessments are also essential.