August 2024
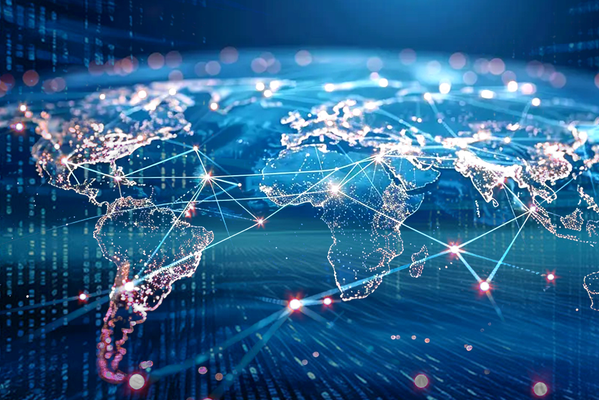
Regulators and policymakers are facing challenges posed by AI-generated content, such as deepfakes creating non-consensual imagery and bots spreading disinformation. To differentiate between synthetic and human-generated content, various approaches are being developed, including AI watermarking, content provenance, retrieval-based detectors, and post-hoc detectors. AI watermarking, in particular, has gained attention, but it lacks standardization and raises privacy concerns. Different jurisdictions are tackling the issue differently, with the USA mandating watermarks on AI-generated material and the EU imposing mandatory disclosures, while China and Singapore require prominent marking and technical solutions like watermarking. Holistic AI offers technical assessments that can help organizations stay ahead of regulatory changes.
April 2024
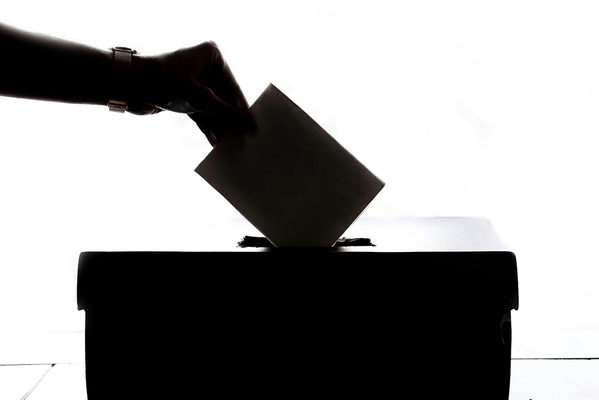
The increase of AI technology in the election process has raised concerns about the potential use of misinformation and deepfakes to manipulate public opinion. Governments and tech companies have taken measures to prevent the spread of AI-generated content, including passing laws requiring disclaimers for AI-generated political advertisements and implementing guidelines for tech platforms to mitigate risks related to elections. However, the efficacy of these measures remains uncertain. Tech giants have also joined forces to combat AI-generated election disinformation, but their agreement lacks binding requirements. Clear disclosures and watermarking are potential safeguards in the ongoing struggle against AI-driven misinformation.
March 2024
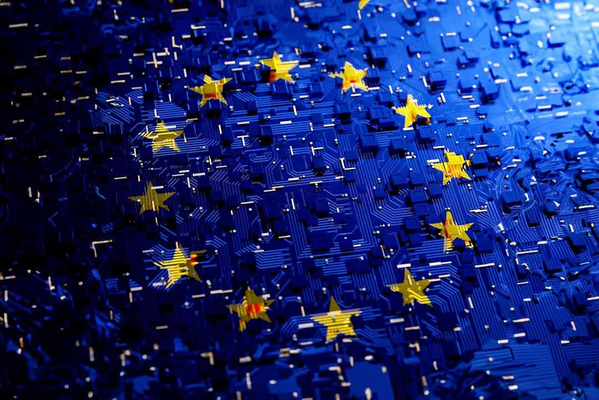
Generative AI is a rapidly expanding field of AI technology that involves creating new content (such as images, text, audio, or other forms of synthetic content) using large datasets and complex algorithms. However, with the enactment of the EU AI Act, generative AI developers are now subject to strict regulatory scrutiny that imposes transparency obligations and additional requirements for high-risk or general-purpose AI models. These obligations include labeling artificially generated content, disclosing deep fake and AI-generated text content, informing natural persons of the existence of AI systems, and complying with copyright laws. Generative AI developers must carefully evaluate and adapt to these requirements to maximize compliance with the EU AI Act.
February 2024
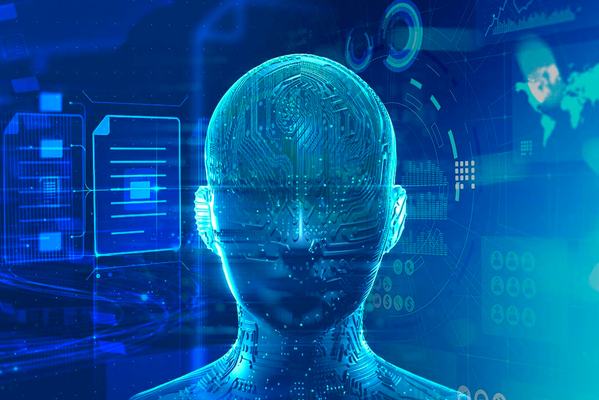
Ensuring the integrity, safety, security, and reliability of generative AI models is crucial for organizations developing and deploying them. Two important processes to achieve this are model evaluations and algorithm audits. While model evaluations assess a model's efficacy across various parameters, such as performance levels and risks, algorithm audits involve independent third-party assessments of reliability, risk detection, and regulatory compliance. Both processes should be used jointly to build the evidence base of a model's safety and risk mitigation capabilities. As regulatory momentum to legislate generative models accelerates, companies must proactively ensure they fulfill their obligations. Holistic AI's LLM Auditing product is a solution that can help identify and address issues such as blocking serious risks, detecting hallucinations and stereotypes, preventing offensive language and toxicity, and providing readability scores.
September 2023
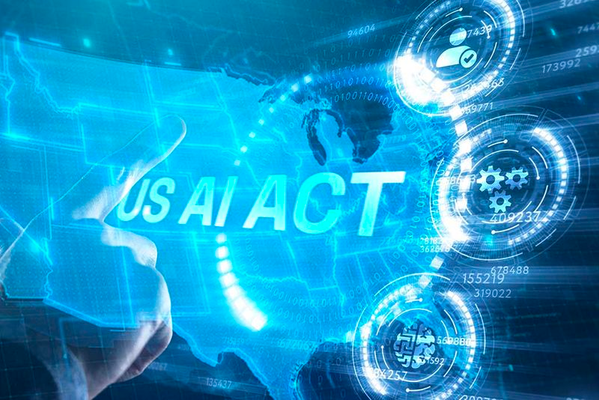
The U.S. Senate Subcommittee on Privacy, Technology, and the Law held a hearing titled "Oversight of AI: Legislating on Artificial Intelligence" to discuss the need for regulation of AI. Senators Blumenthal and Hawley announced a bipartisan legislative framework to address five key areas: establishing a licensing regime, legal accountability for harms caused by AI, defending national security and international competition, promoting transparency, and protecting consumers and kids. The hearing also addressed the need for effective enforcement, international coordination, and protecting against election interference, surveillance, and job displacement. Compliance requirements for companies using AI are expected to evolve with the new AI regulations.