July 2023
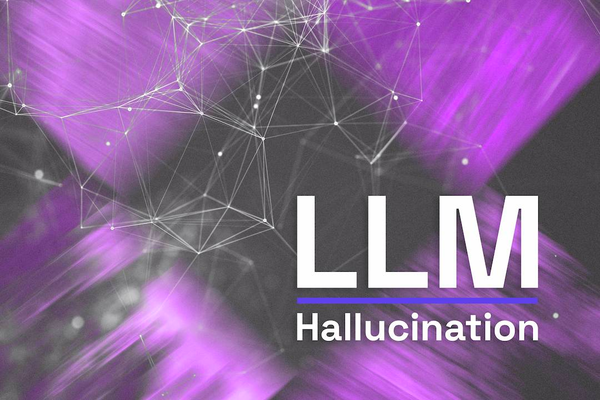
Large language models (LLMs) can produce hallucinations, which are inaccuracies in the information generated that are neither present in nor correctly inferred from their training data. There are four primary categories of hallucinations, including dialogue history-based hallucinations, hallucinations in abstractive summarisation, generative question answering hallucinations, and general data generation hallucinations. The root cause of hallucinations is the probabilistic nature of LLMs, which lack common sense or factual knowledge and instead rely on pattern recognition and statistics. Holistic AI offers innovative solutions and an AI risk management platform to help organizations navigate the complex terrain of AI and harness the power of LLMs with confidence and efficiency. This blog article is not intended to provide legal advice or opinion and is not a substitute for experienced legal counsel.
May 2023
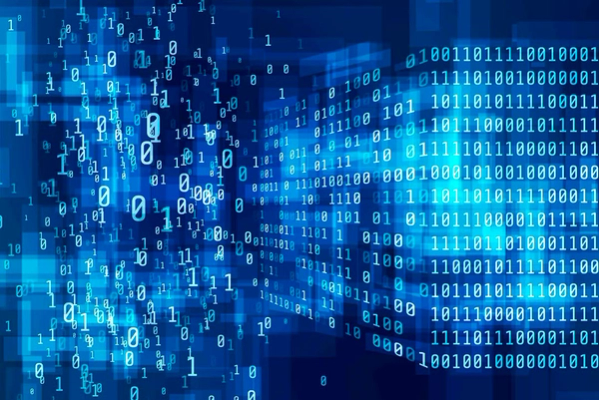
Large Language Models (LLMs) have come a long way, with pre-trained artificial intelligence models serving as a foundational base for a wide variety of applications and tasks. LLMs have immense potential, but it is essential to remain aware of equity, fairness, and ethical issues they may present, as well as the limitations they must overcome to develop Artificial General Intelligence (AGI). The widespread adoption of LLMs needs to be balanced with addressing potential risks to society and humanity. Data is becoming a significant constraint for optimal LLM performance, necessitating innovative approaches to balance model size and training tokens. Innovative approaches are currently addressing the limitations of current language models, providing alternative solutions to data constraints. Together, these strategies offer a promising path to overcome data constraints and improve the effectiveness and versatility of language models.
February 2023
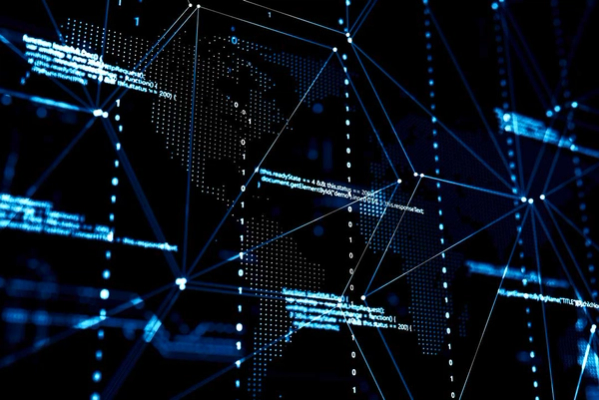
AI-based conversational agents such as ChatGPT and Bard have become increasingly popular and are entering our daily lives through browsers and communication platforms. The key to staying ahead is to be aware of the new technology trends, particularly the innovative Transformer architecture of deep learning models that has redefined the way we process natural language text. Language modelling approaches such as Masked Language Modeling (MLM) from BERT and Causal Language Modeling (CLM) from GPT have represented a significant leap forward in NLP technology, but each model has its limitations. To make language models more scalable for commercial solutions, researchers and engineers have sought a new approach such as InstructGPT and LaMDA. These technologies use fine-tuning and reinforcement learning strategies applied to human feedback to meet the user's requests more accurately.
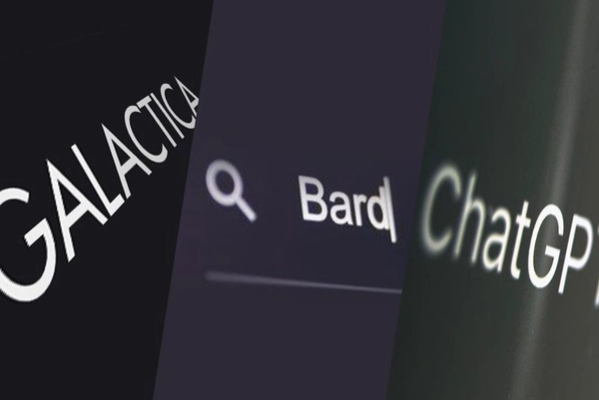
Large language models (LLMs) such as Galactica, ChatGPT, and BARD are gaining popularity and being integrated into various aspects of daily life. While they have many benefits, society must understand their limitations, biases, and regulatory issues. The use of LLMs raises concerns about responsibility for generated responses and measuring/mitigating bias and discrimination in the models. Regulation could include data representativeness audits and human evaluations to ensure fair and accurate results. It is essential to comprehend the workings of LLMs and put governance mechanisms in place to address associated risks.