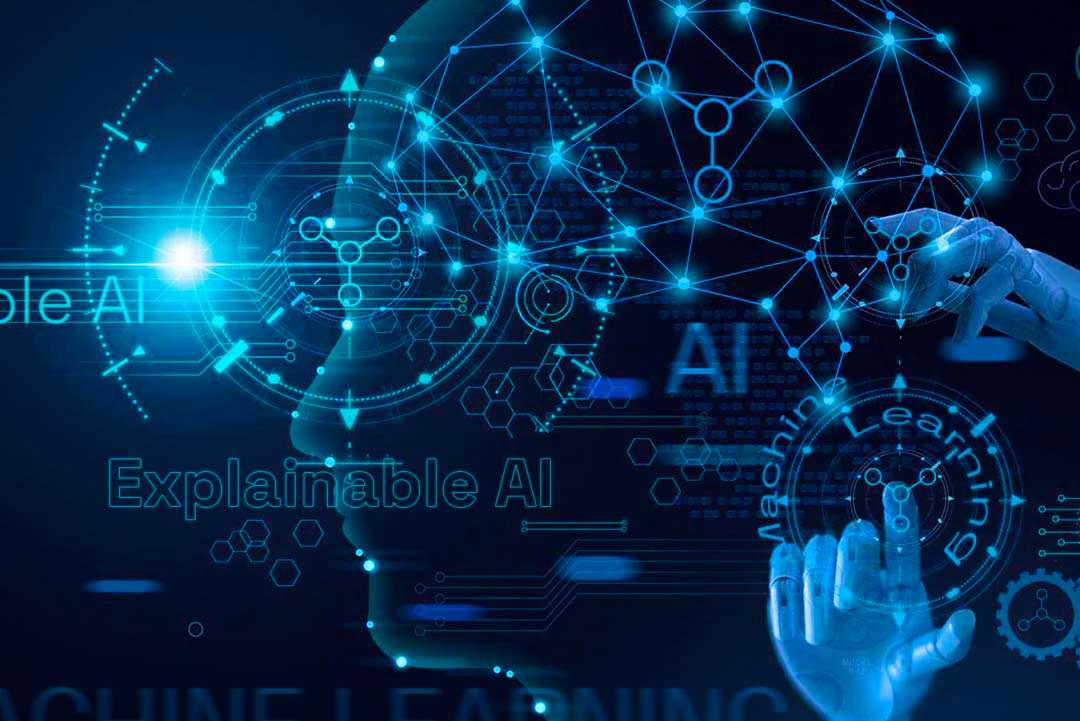
Explaining Machine Learning Outputs: The Role of Feature Importance
Kleyton da Costa
04 Aug 2023
In an age where artificial intelligence permeates nearly every aspect of our lives, the inner workings of these intelligent systems often remain shrouded in mystery. However, with the rise of explainable AI (XAI), a groundbreaking paradigm is transforming the AI landscape, bringing transparency and understanding to complex machine learning models. Gone are the days of accepting AI decisions as enigmatic black-box outputs; instead, we are now entering an era where we can uncover the underlying rationale behind AI predictions.
In this post, let's briefly introduce two strategies for global feature importance – permutation feature importance and surrogacy feature importance. Additionally, we'll start with some important definitions to understand and categorise the topics that make up the field of explainable AI.
Continue reading on
Holistic
AI Tracker
Create your account
Create a FREE account and access a number of articles, resources and guidance information.
Already have an account? Log In