January 2023
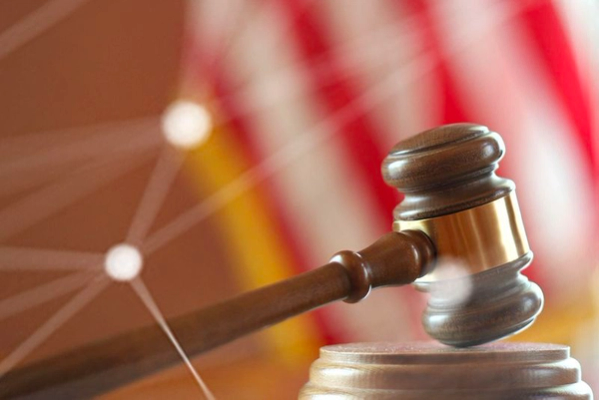
The US Equal Employment Opportunity Commission (EEOC) has published a Strategic Enforcement Plan (SEP) for the 2023-2027 fiscal years, which prioritizes the regulation of AI and automated employment tools to prevent discrimination against protected groups. The EEOC aims to ensure that these tools do not disproportionately impact protected subgroups, and has launched initiatives to examine the impact of AI on employment decisions. The EEOC recently sued iTutorGroup for age discrimination due to their use of software to reject older applicants, highlighting the importance of regulation in preventing AI-related discrimination in employment.
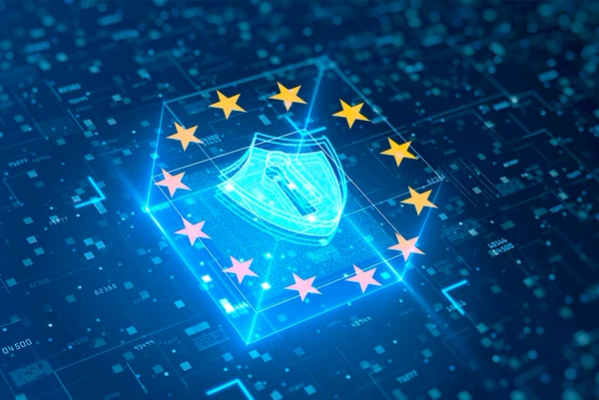
The Digital Markets Act (DMA) came into effect on 1 November 2022 and focuses on regulating fair competition and consumer choice in the digital economy. The legislation defines gatekeepers as providers of core platform services and imposes specific obligations, including an independent audit on companies designated as gatekeepers. The DMA will be enforced from February/March 2024 and failure to comply could result in fines of up to 10% of the company’s total worldwide annual turnover. The DMA, in combination with the Digital Services Act (DSA) and the EU AI Act, is set to make digital technologies safer for users and companies will struggle to find loopholes to avoid doing their due diligence. The DMA is anticipated to set a global precedent, and regulation like this will soon mean that AI around the world is deployed more transparently and with greater accountability.
December 2022
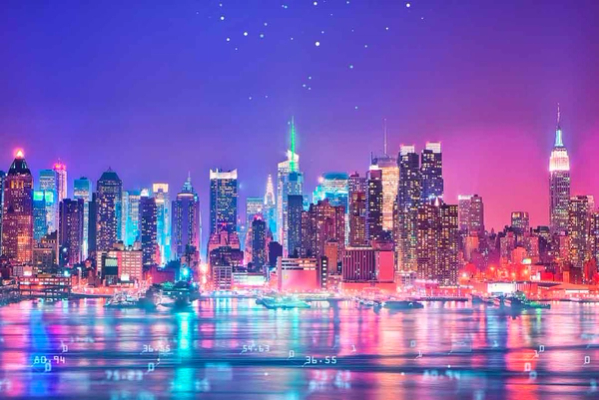
New York City's Local Law 144 mandates the use of independent impartial bias audits for automated employment decision tools (AEDTs) used for employment or promotions. The enforcement date has been pushed back to 2023 due to concerns about who qualifies as an independent auditor and the suitability of the impact ratio metrics. The updated rules clarify that bias audits must be conducted by a third party and include metrics for calculated impact ratios based on selection rate or average score. The audit can be based on test data when historical data is not available. Additionally, employers must provide AEDT data retention policies, making them available on their website. Holistic AI offers auditing services for businesses seeking compliance.
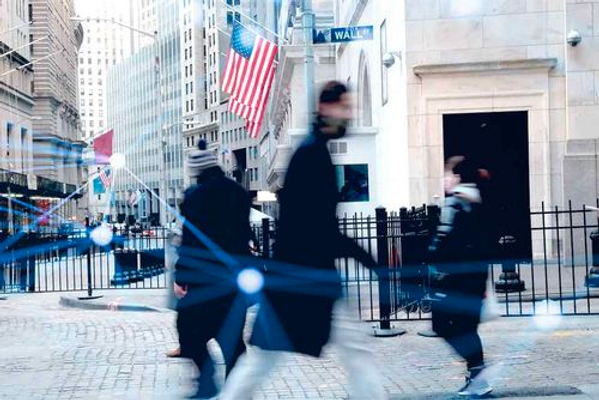
The New York Department of Financial Services published a circular letter in January 2019 to insurers authorized to write life insurance in the state. The letter warns insurers not to use external data sources, algorithms, or predictive models in underwriting or rating unless it has been determined that the system does not collect or use prohibited criteria. The burden and liability lie with the insurer, and the NYDFS reserves the right to audit and examine an insurer’s underwriting criteria, programs, algorithms, and models and can take disciplinary action if necessary. The letter also highlights the obligation to comply with existing anti-discrimination and civil rights laws and regulations. Insurers should provide transparency to consumers regarding the reason or reasons for any adverse underwriting decisions made using external data sources or predictive models. Failure to comply may result in an NYDFS audit and breach of existing anti-discrimination laws.
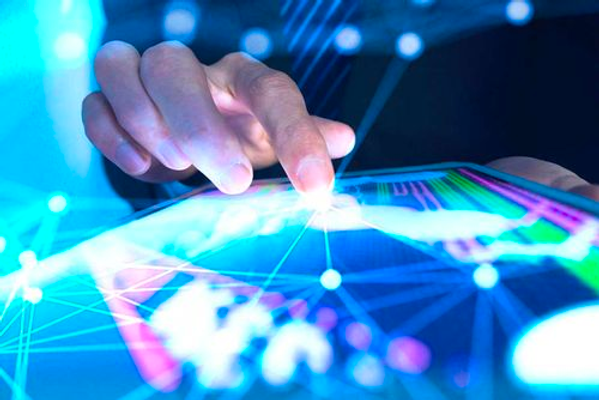
21 Dec 2022
The article discusses the current regulatory environment surrounding artificial intelligence (AI) and the need for AI auditing to ensure the safety, legality and ethics of AI systems. The process of AI auditing involves four stages, including triage, assessment, mitigation, and assurance. The assessment phase evaluates the efficacy, robustness and safety, bias, explainability, and algorithm privacy of the system. The audit outcomes are used to inform the residual risk of the system, and mitigation actions are suggested to address the identified risks. The importance of conducting an audit of an AI system is highlighted, including improving stakeholder confidence and trust and future-proofing systems against regulatory changes.