December 2024

19 Dec 2024
AI agents are autonomous systems built on large language models that can make judgments and take actions on behalf of users or other systems. They interact with users and their environment and have the ability to access external tools to make decisions. AI agents can learn and improve over time, and have various uses in enterprise settings such as customer service, process automation, and marketing. However, they face challenges such as complexity, resource-intensiveness, privacy concerns, and the need for human oversight during development stages. Additionally, there are ethical concerns associated with AI agents' ability to replicate human personalities, potentially leading to privacy violations and deepfake-related issues. Holistic AI's Safeguard can help audit and monitor LLMs for safer and faster deployment of AI tools.
August 2023
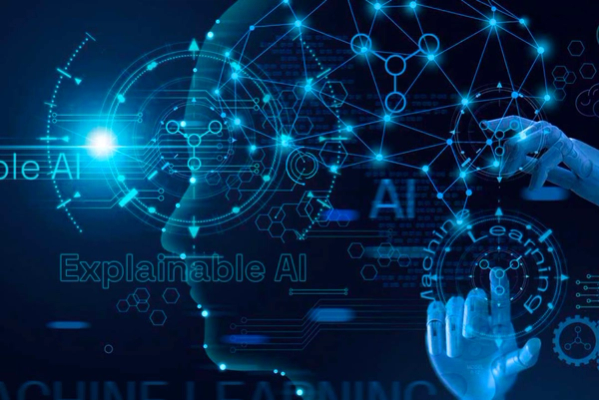
Explainable AI (XAI) is a new paradigm in AI that brings transparency and understanding to complex machine learning models. Two strategies for global feature importance in ML models are permutation feature importance and surrogacy feature importance. Permutation feature importance involves systematically shuffling the values of a single feature while keeping the other set of features unchanged to observe how the shuffling impacts the predictive accuracy or performance metric of the model. Surrogacy feature importance relies on creating interpretable surrogate models to gain insights into complex black box models. These techniques enable stakeholders to trust the predictions of the model and make informed decisions based on the model's output, leading to a culture of transparent and trustworthy AI systems. Holistic AI is a company that helps organizations validate their machine learning-based systems to allow safe, transparent, and reliable use of AI.
June 2023
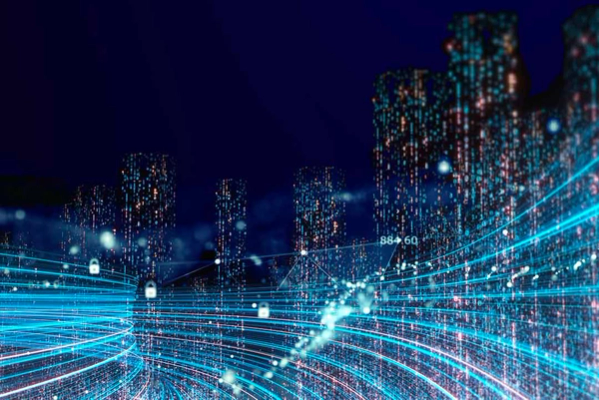
The article discusses the varying definitions of Artificial Intelligence (AI) and its impact on society, including real-world examples of AI applications in industries such as healthcare and finance. It highlights the benefits and risks of AI, including ethical concerns about job displacement, privacy, and AI-proliferated misinformation. The article also explores the future of AI, including generative AI and the theoretical concept of artificial general intelligence that could one day solve the world's most complex problems. The article stresses the importance of understanding the applications and impact of AI and engaging in ethical discussions around bias and responsible deployment.
April 2023
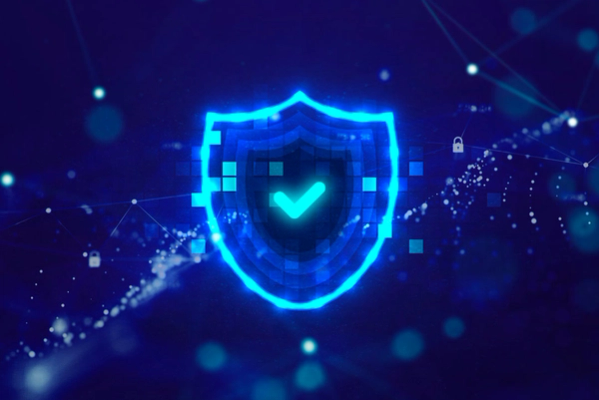
There has been a discussion about the need for a pause in using generative artificial intelligence but it is not practical as AI models are already used in various aspects of daily life. It is essential to approach AI with a nuanced understanding of potential benefits and risks, and prioritize responsible and ethical practices. Fairness, bias mitigation, model transparency, robustness, and privacy are crucial elements that increase trust in AI systems and contribute to a more trustworthy ecosystem. Consumers value companies that adopt responsible AI policies, making it essential for companies to prioritize responsible and ethical AI practices to enhance their brand reputation and contribute to a more trustworthy AI ecosystem. Continued research and collaboration between researchers, policymakers, and stakeholders are necessary to create more responsible and transparent AI systems, address potential risks, and ensure that AI is developed and deployed ethically.
March 2023
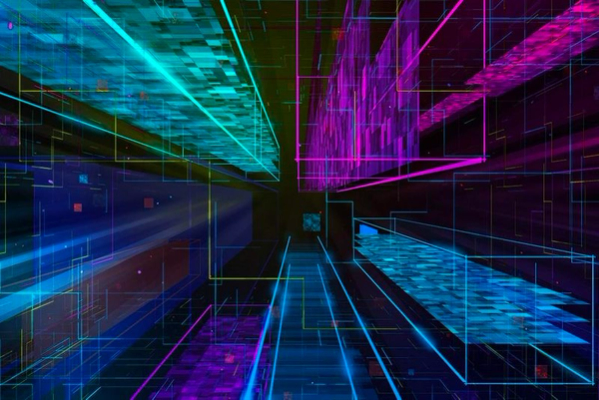
In critical areas such as healthcare and self-driving cars where AI is being increasingly used, the efficacy of algorithms is crucial. The measurements of efficacy depend on the type of system and its output. Classification systems rely on metrics such as true and false positives and negatives, accuracy, precision, recall, F1 scores, and area under the operating curve. For regression systems, correlations and root mean square error are used to compare outputs with ground truth scores. The choice of metric depends on the context and type of model being used. Holistic AI's open-source library provides built-in metrics for measuring model performance.