August 2022
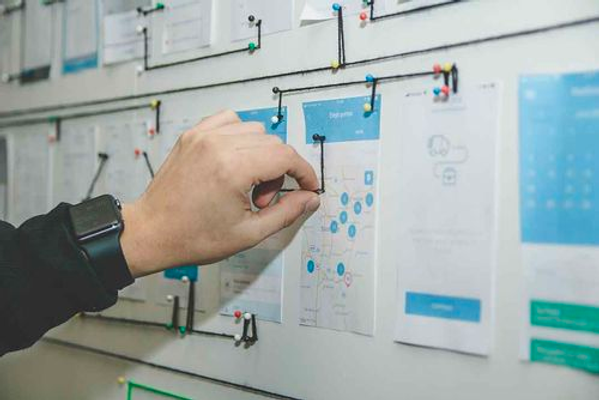
The US National Institute of Standards and Technology (NIST) has published a second draft of its AI Risk Management Framework (AI RMF), which explains how organizations should manage the risks of AI. The AI RMF is a set of voluntary guidelines to help prevent potential harms to people, organizations, or systems resulting from AI systems' development and deployment. The framework has four core elements - govern, map, measure, and manage - that aim to cultivate a culture of AI risk management, establish appropriate structures, policies and processes, understand the AI system's business value, and assess risks with bespoke metrics and methodologies. NIST expects AI risk management to become a core part of doing business by the end of the decade, just like privacy and cybersecurity.
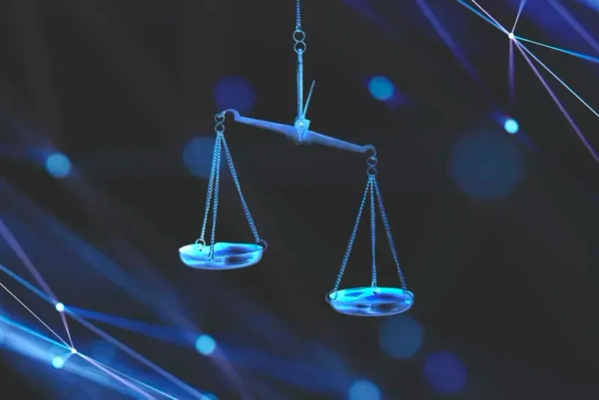
To address concerns regarding the use of artificial intelligence (AI), regulations have been developed. There are two approaches to regulating AI: horizontal and vertical. Horizontal regulation applies to all applications of AI across all sectors and is typically controlled by the government, while vertical regulation only applies to a specific application of AI or sector and may be delegated to industry bodies. Each approach has its pros and cons, such as flexibility, standardization, and coordination. Examples of horizontal regulation include the EU AI Act and the US Algorithmic Accountability Act, while examples of vertical regulation include the NYC bias audit mandate and the Illinois Artificial Intelligence Video Interview Act. It is essential to note that this article does not offer legal advice.
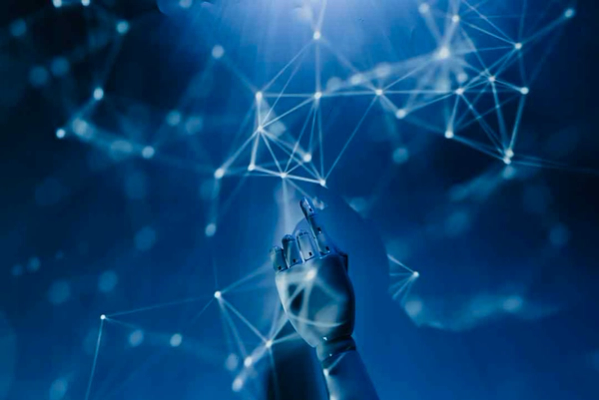
The use of algorithms and automation brings about many benefits, but it also poses risks, as shown in high-profile cases of harm associated with their use. Applying AI ethics principles could have prevented these harms from occurring. Cases that highlight the risks associated with algorithms include the COMPAS tool, Amazon's scrapped resume screening tool, and Apple's algorithm used to determine credit limits. AI ethics principles could have been useful in mitigating these issues if bias assessments and checks for differential accuracy for subgroups were conducted. It stresses the importance of transparency and explainability when it comes to automated decision tools and the assurance of algorithms to reduce the harm that can result from their use.
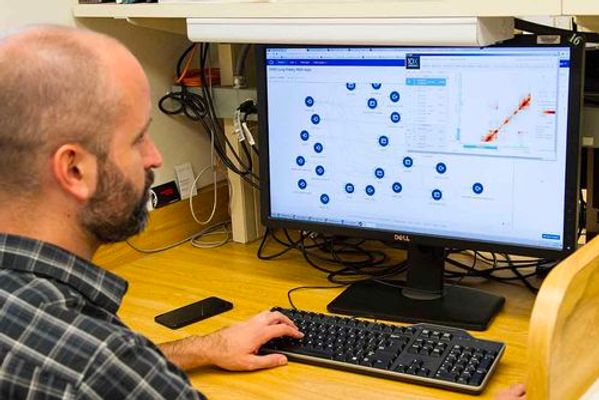
Impact assessments, including Algorithmic Impact Assessments (AIAs) and Data Protection Impact Assessments (DPIAs), can be used to determine the potential risks and harm associated with a system. AIAs are used to identify the risks associated with the use of an automated decision system, while DPIAs are used to assess data management strategies. These assessments are increasingly being used to determine the impact of AI systems on users and stakeholders. EU AI Act requires AIAs to determine significant risks posed by high-risk systems to fundamental rights, health, or safety. A higher impact system is subject to tighter regulations and stricter requirements, and low impact systems are subject to more lenient requirements.
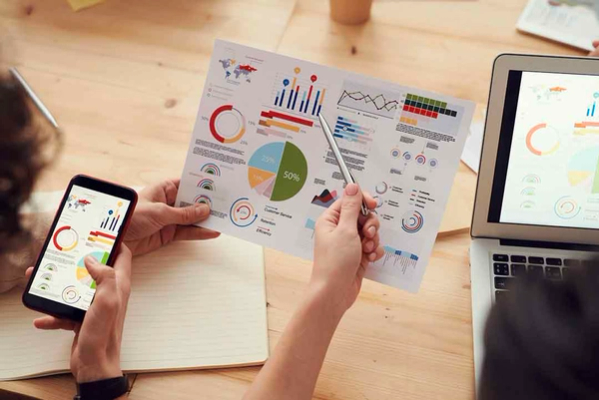
The rise in AI use has led to concerns about its legal, ethical, and safety implications, resulting in the emergence of AI ethics. Algorithm auditing is an approach to mitigating potential harm that can arise from high-risk AI systems such as those in healthcare, recruitment, and housing. Auditing involves assessing an algorithm's safety, ethics, and legality and consists of five stages of development: data and set up, feature pre-processing, model selection, post-processing and reporting, and production and deployment. Following the audit, assurance is the process of determining whether the AI system conforms to regulatory, governance, and ethical standards, including certification, impact assessments, and insurance. Whilst auditing contributes to assuring an algorithmic system, governance and impact assessments are also essential.