March 2023
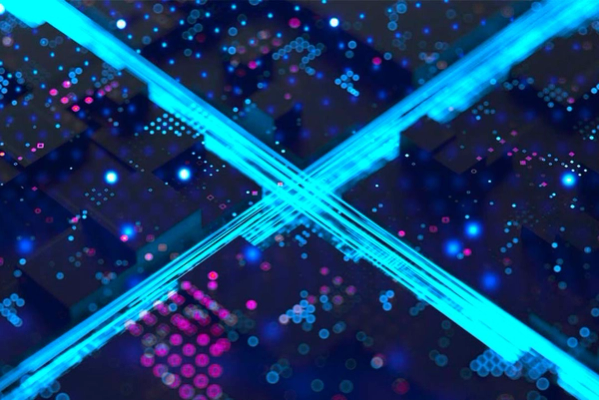
Explainable AI has become increasingly important due to the need for transparency and security in AI systems. The SHAP model, inspired by game theory, is widely used for interpreting machine learning models. The SHAP algorithm calculates the contribution of each feature to the final prediction made by the model, allowing for local and global explanations. The assumptions of model-agnostic approximation methods simplify the calculation of explanations, but may not hold true for all models. The article demonstrates the interdisciplinary nature of explainable AI and its potential to identify and correct errors and bias, as well as ensure that AI models comply with ethical and legal standards.
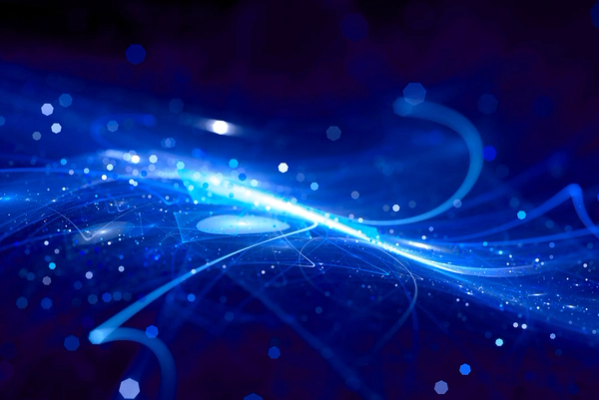
The challenge with artificial intelligence (AI) models is the trade-off between accuracy and explainability. The more accurate the results, the less understandable they are to humans. Therefore, explainable AI has gained importance, which refers to the ability to explain the decisions of an AI model in humanly understandable terms. The article discusses the practical and societal dimensions of explainable AI. In the practical dimension, engineers and data scientists need to implement explainable solutions in their models, and end-users must comprehend the AI-generated outcomes to make informed decisions. In the societal dimension, explanations need to be provided to all relevant stakeholders to ensure that AI systems' decisions are fair and ethical. Finally, explainability is crucial for AI risk management, enabling auditors to understand how AI models arrive at their decisions and ensure they are ethical, transparent, and bias-free.
February 2023
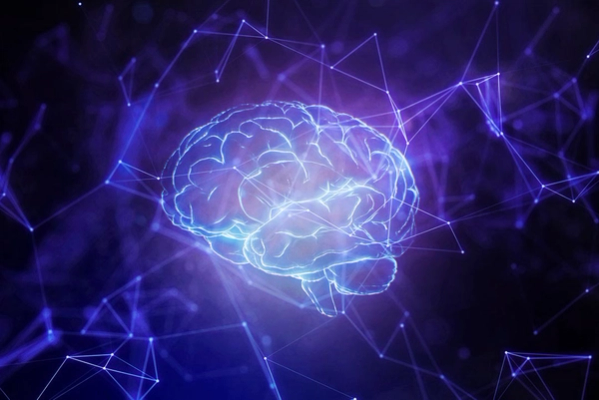
06 Feb 2023
The increasing integration of artificial intelligence (AI) in various aspects of our lives requires transparency around the data the systems use to generate outputs, and that the decisions made are explainable and their implications communicated to relevant stakeholders. AI transparency comprises three levels: explainability of the technical components, governance of the system, and transparency of impact. The goal of AI transparency is to establish an ecosystem of trust around the use of AI, particularly among citizens or users of systems, and especially in communities that are at the most risk of harm by AI systems. AI transparency and explainability can build trust in AI systems, give individuals more agency over their decisions, and have several business benefits.
January 2023
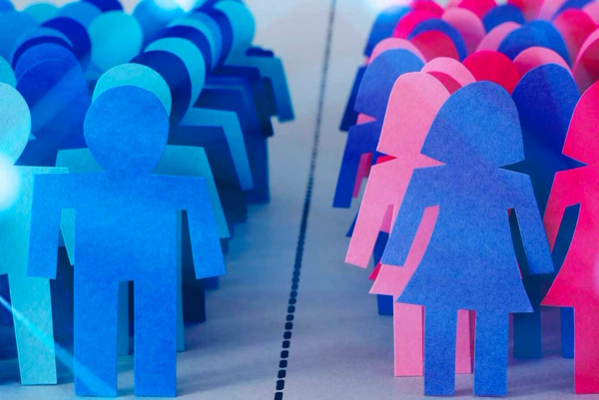
New York City passed Local Law 144 in November 2021 to mandate bias audits of automated employment decision tools (AEDTs) used in candidate screening and promotion. The Department of Consumer and Worker Protection (DCWP) proposed metrics to calculate impact ratios for regression systems, but they have limitations, such as being fooled by unexpected distributions and data tweaking. The article suggests using different metrics that consider fairness over the whole distribution, tests to compare different distributions, or metrics that compare the ranking of candidates rather than the score itself. Holistic AI offers an open-source library of metrics for both binary and regression systems and bias mitigation strategies.
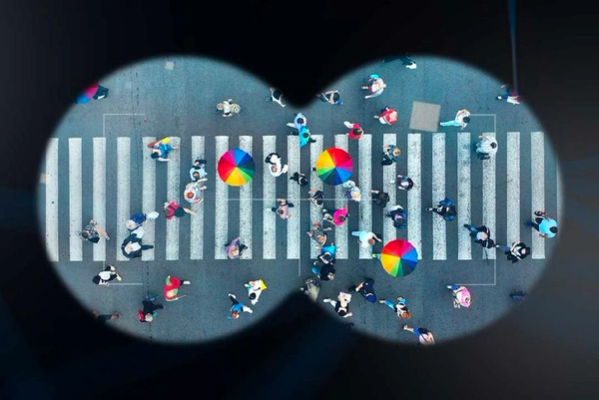
New York City has passed a new law called Local Law 144 requiring employers and employment agencies to commission independent, impartial bias audits of automated employment decision tools (AEDTs) being used when evaluating candidates for employment or employees for promotion. The bias audits will be based on impact ratios using the Equal Employment Opportunity Commission's four-fifths rule to calculate whether a hiring procedure results in adverse or disparate impact. However, the rule can provide false positives when sample sizes are small, and the NYC legislation doesn't provide guidance on this issue. The enforcement date of Local Law 144 has been delayed to July 5, 2023, providing employers, employment agencies, and vendors more time to collect additional data and make the analysis more robust.