October 2024
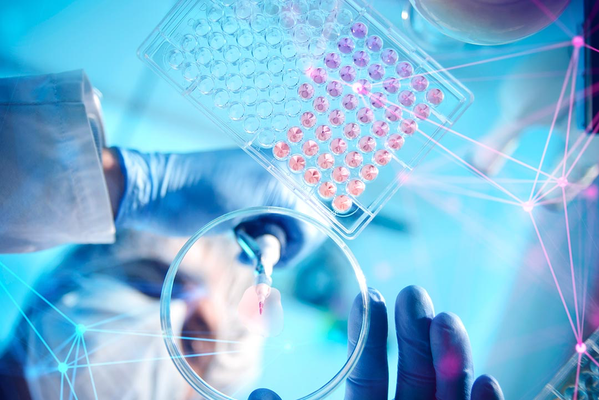
Healthcare systems are using digital technologies, resulting in large amounts of data that can be analyzed by machine-learning algorithms to aid in diagnosis, prognosis, triage, and treatment of diseases. However, the translation of these algorithms into medical practice is hindered by a lack of careful evaluation in different settings. Guidelines for evaluating machine learning for health (ML4H) tools have been created to assess models for bias, interpretability, robustness, and possible failure modes. This study applied an ML4H audit framework to three use cases, which varied in findings but highlighted the importance of case-adapted quality assessment and fine-grained evaluation. This paper suggests improvements for future ML4H reference evaluation frameworks and discusses the challenges of assessing bias, interpretability, and robustness. Standardized evaluation and reporting of ML4H quality are essential to facilitate the translation of machine learning algorithms into medical practice.
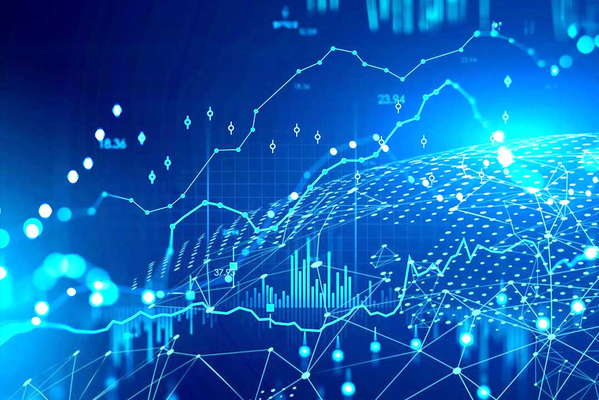
The US Department of State has published a Risk Management Profile for Artificial Intelligence and Human Rights, a voluntary guidance aimed at providing guidelines for governmental, private, and civil society entities on AI technologies, consistent with international human rights. The profile uses the National Institution of Standard and Technology's AI Risk Management Framework 1.0 that provides an AI lifecycle risk management strategy approach and examples of common organizational functions. The Profile is not exhaustive, yet it provides possible human rights implicating situations that organizations may encounter when using AI systems. It is a normative rationale for adopting the US’s approach to AI governance and risk mitigation strategies that will drive long-term considerations in this arena.
July 2024
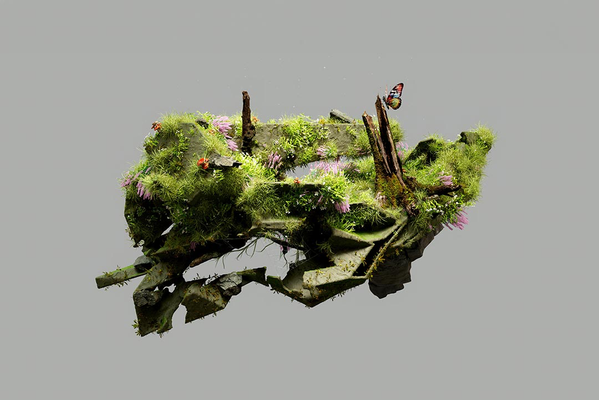
The "green transition" towards renewable energy and industrial processes will require a significant increase in the consumption of metals and minerals, which will involve expanding mining operations. The article explores the use of AI to extract valuable resources and mitigate the negative impacts of mining on local communities. AI can aid companies and governments in monitoring mine site violations, issues in mineral processing, and raw material supply chains. An interactive Multi Objective Optimization (iMOO) approach that captures the linkages between investment decisions and environmental, social, and economic outcomes is being developed and piloted. By including outcomes along environmental and social criteria, iMOO can promote more sustainable decision making, while allowing for the consideration of complex data and its linkages. However, information equality and the transparency of those results and access to these tools for companies, communities, and governments is critical. Computing the Pareto fronts that include social and environmental sustainability criteria helps communication among decision-makers and can help in addressing ethical concerns around AI use.
June 2024
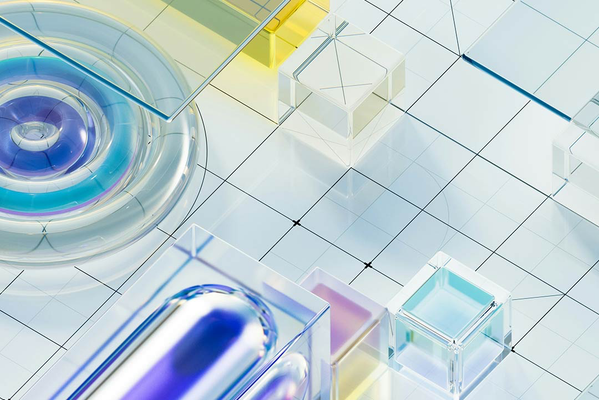
The adoption of artificial intelligence (AI) in financial services, particularly in syndicated lending, can offer benefits such as reducing costs, improving documentation production and completion, and improving risk management. However, lenders and other market participants adopting AI must consider the regulatory landscape across different jurisdictions, which can vary in their approaches to regulating AI. To operationalize the use of AI in a compliant manner, institutions must develop internal guidelines encompassing AI governance policy, AI model lifecycle management policy, policy on compliant and ethical use of AI and risk identification and management policy. While concerns exist about the impact of AI on jobs, its adoption in syndicated lending can ultimately help employees focus on higher-value tasks.
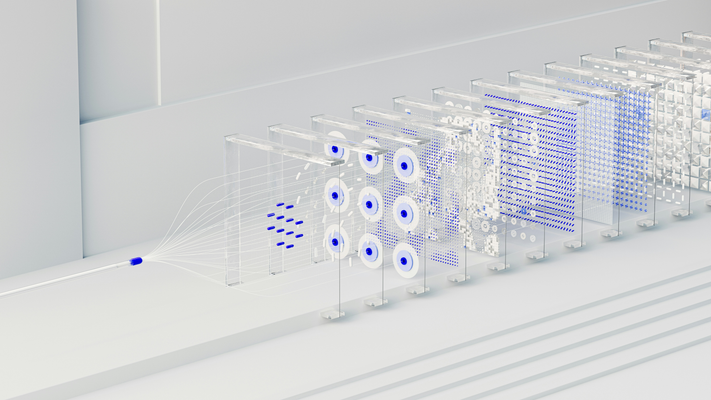
Artificial general intelligence (AGI) has the potential to revolutionize science and technology, but responsible management is crucial to ensure that it aligns with human values and does not harm human interests. AI alignment focuses on the internal workings of AI systems, while AI governance focuses on the broader regulatory and policy framework governing AI’s integration into society. Misalignment can bring various risks, including safety, ethical, economic, employment, and security risks. Several approaches to AI alignment address different aspects of the problem, with varying degrees of effectiveness and scalability. The major challenge of AI alignment is scalable alignment, which requires evolving methodologies to manage increasingly complex and capable AI systems while preserving human control and promoting their utilization for societal benefit.