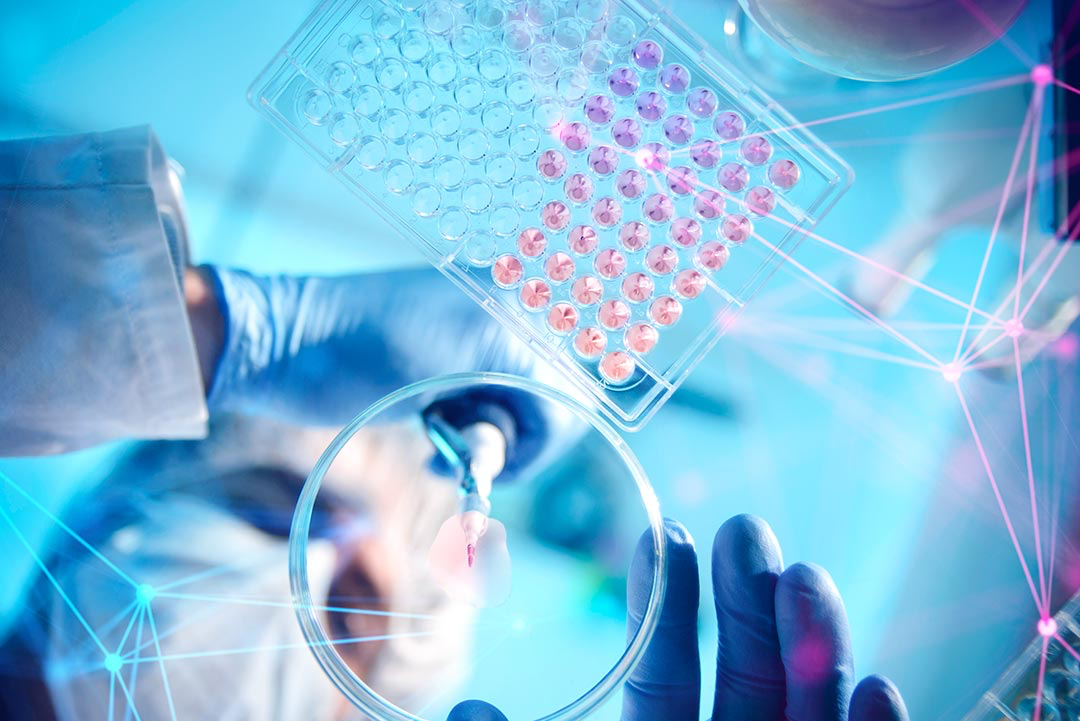
ML4H Auditing: From Paper to Practice
Giovanna Jaramillo-Gutierrez
23 Oct 2024
Healthcare systems are currently adapting to digital technologies, producing large quantities of novel data generated from medical imaging, sensors, or electronic health records. Based on these data, machine-learning algorithms have been developed to support practitioners in labor-intensive workflows such as diagnosis, prognosis, triage or treatment of disease. Modern machine learning technology has been developed to analyze big data for health, promising to reduce cost and labor for diagnostics and prognostics in different medical fields (Topol,2019; Esteva et al., 2019). However, their translation into medical practice is often hampered by a lack of careful evaluation in different settings. Efforts have started worldwide to establish guidelines for evaluating machine learning for health (ML4H) tools, highlighting the necessity to evaluate models for bias, interpretability, robustness, and possible failure modes. A sprawling ecosystem comprising academic, corporate, and institutional capital has produced numerous ML4H use cases such as detecting diabetic retinopathy in retina images (Gulshan et al., 2016) or predicting Alzheimer’s disease from MRI images (Moradi et al., 2015). The exploratory excitement is matched by demands for a rigorous assessment of efficacy and safety as is standard protocol for any technological innovation in healthcare, giving rise to a colorful smorgasbord of initiatives creating guidelines for transparent assessment of ML4H performance, such as STARD-AI (Sounderajah et al., 2020), CONSORT-AI (Liu et al., 2020), SPIRIT-AI (Liu et al., 2020) and the World Health Organization (WHO)/International Telecommunication Union (ITU) Focus Group on Artificial Intelligence for Health (FG-AI4H) (Wiegand et al., 2019). Integrating these guidelines into the machine learning development process to meet technical, ethical, and clinical requirements is challenging. While there appears to be no shortage in good practice guidelines on paper, the question on how well they can be adopted in practice remains unanswered.
Continue reading on
Holistic
AI Tracker
Create your account
Create a FREE account and access a number of articles, resources and guidance information.
Already have an account? Log In