March 2023
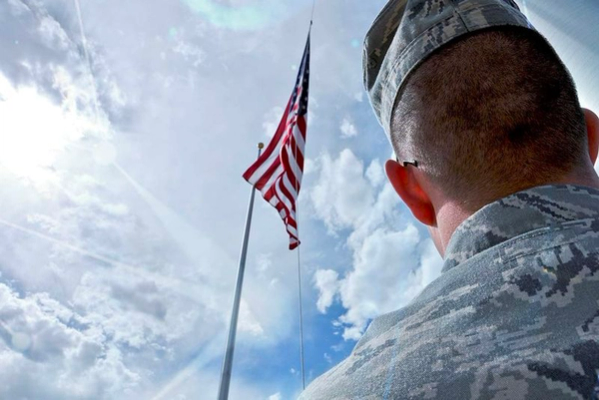
The US Department of State has released a "Political Declaration on Responsible Military Use of Artificial Intelligence and Autonomy", outlining 12 best practices for responsible AI in military applications. The declaration emphasizes the importance of using AI in accordance with international law and developing auditable methodologies to avoid unintended consequences and bias. It has been signed by 60 countries, including the US and China. The US Department of Defense adopted its own Ethical Principles for Artificial Intelligence in 2020, with the aim of facilitating the lawful use of AI systems in both combat and non-combat functions. The Department's approach to Responsible Artificial Intelligence largely focuses on supplementing existing laws, regulations, and norms to address novel issues stemming from AI, with a focus on reliability, risk management, and ethics.
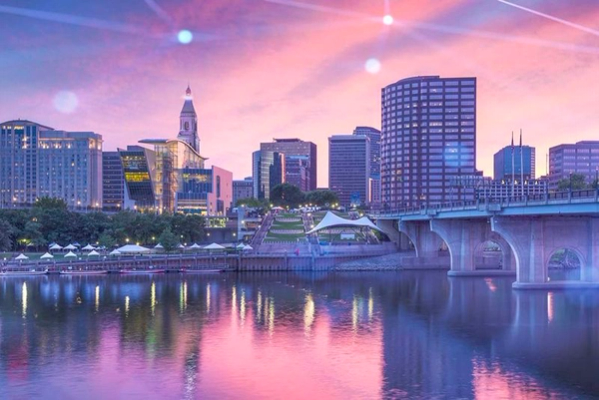
Connecticut lawmakers have proposed a bill that would establish an Office of Artificial Intelligence and a government task force to develop an AI Bill of Rights. The bill would require government oversight, mandate inventory and testing of state-used algorithms, close existing data privacy loopholes, and enumerate citizen protections through an AI Bill of Rights. The bill seeks to regulate the use of AI by state agencies and outlines protocols and processes for developing, procuring, and implementing automated decision systems. The Office of Artificial Intelligence would conduct periodic re-evaluations of automated systems to ensure compliance with these protocols. The bill emphasizes the necessity for governments to stay current with AI regulations to safeguard individuals from potential harm.
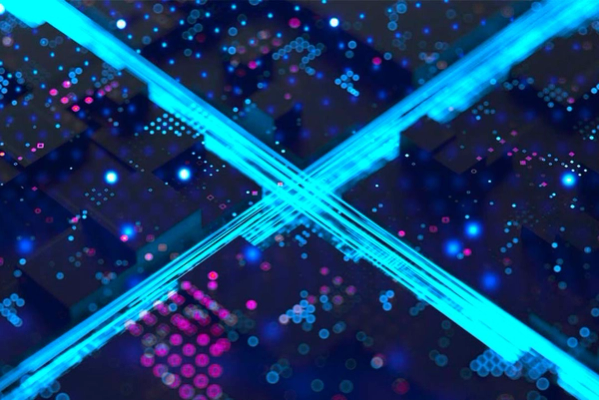
Explainable AI has become increasingly important due to the need for transparency and security in AI systems. The SHAP model, inspired by game theory, is widely used for interpreting machine learning models. The SHAP algorithm calculates the contribution of each feature to the final prediction made by the model, allowing for local and global explanations. The assumptions of model-agnostic approximation methods simplify the calculation of explanations, but may not hold true for all models. The article demonstrates the interdisciplinary nature of explainable AI and its potential to identify and correct errors and bias, as well as ensure that AI models comply with ethical and legal standards.
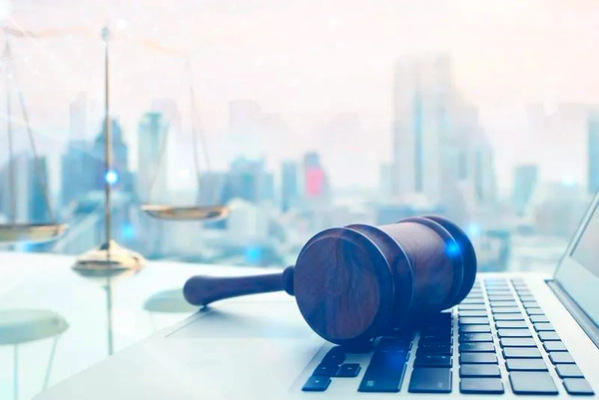
Governments and public sector entities are increasingly using artificial intelligence (AI) to automate tasks, from virtual assistants to defense activities. However, there are risks associated with AI use, and steps must be taken to reduce these risks and promote safe and trustworthy use. Policymakers worldwide are proposing regulations to make AI systems safer, targeting both AI applications by businesses and government use of AI. The US, UK, and EU have taken different approaches to regulating AI in the public sector, with efforts ranging from guidelines to laws. These include the Algorithm Registers in the Netherlands, the UK's guidelines for AI procurement, and the US's AI Training Act. Compliance with these requirements and principles is necessary for governments and businesses when deploying or procuring AI systems.
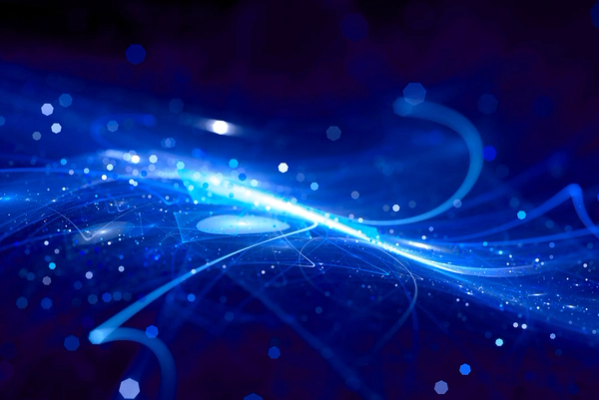
The challenge with artificial intelligence (AI) models is the trade-off between accuracy and explainability. The more accurate the results, the less understandable they are to humans. Therefore, explainable AI has gained importance, which refers to the ability to explain the decisions of an AI model in humanly understandable terms. The article discusses the practical and societal dimensions of explainable AI. In the practical dimension, engineers and data scientists need to implement explainable solutions in their models, and end-users must comprehend the AI-generated outcomes to make informed decisions. In the societal dimension, explanations need to be provided to all relevant stakeholders to ensure that AI systems' decisions are fair and ethical. Finally, explainability is crucial for AI risk management, enabling auditors to understand how AI models arrive at their decisions and ensure they are ethical, transparent, and bias-free.