April 2025
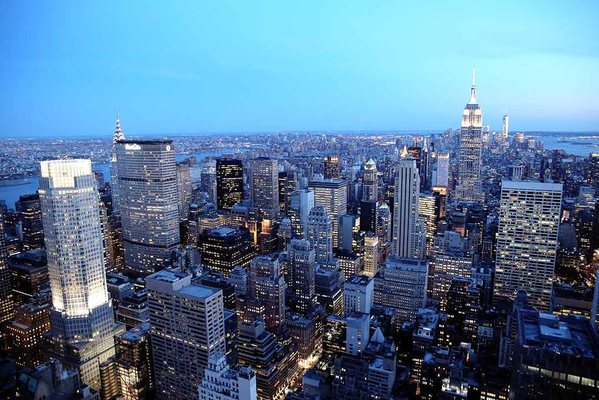
Depending on how they are used, agentic AI powered HR tech tools may require annual, independent bias audits.
February 2025
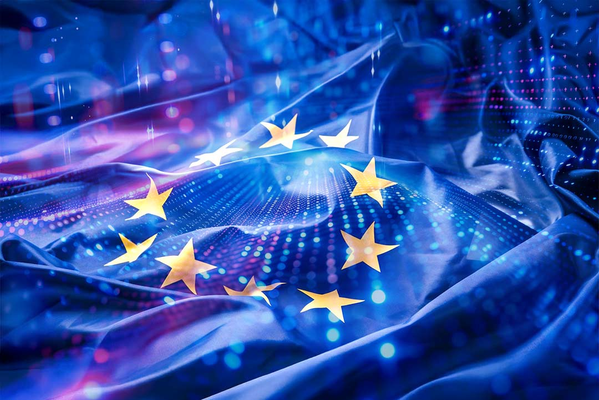
Yesterday, February 2, 2025, the first provisions of the EU AI Act went into effect, including those relating to AI literacy and prohibited systems. Prohibited systems include those that pose an unacceptable level of risk to fundamental rights, health, or safety, and penalties for their use can be up to €35 million or 7% of annual worldwide turnover. Providers and deployers of AI systems must ensure sufficient AI literacy for staff and agents. Compliance requires creating an inventory of AI systems, classifying them, and ensuring ongoing AI literacy training. Holistic AI offers a solution to simplify compliance with the EU AI Act.
January 2025

President Trump signed an executive order in January 2025 to remove barriers to American leadership in artificial intelligence (AI), emphasizing the need to solidify the US' position as a global leader in AI and to develop unbiased AI systems. To achieve its goals, the administration's AI policy vision will be developed and steps will be taken to de-implement President Biden's AI executive order. An AI action plan must be conceptualized and delivered, and the White House's Office of Budget and Management must publish a memorandum implementing the AI executive order by March 25th, 2025. The Trump administration also revoked President Biden's AI executive order and is reviewing federal agency actions taken under it to ensure consistency with the new policy. Despite the revocation of the Biden order, AI innovation and safety remain priorities for the US government.

US President Joe Biden issued an Executive Order (EO) to speed up the development of AI infrastructure in the US, prioritizing economic competitiveness, national security, AI safety, clean energy, and maintaining low energy prices for consumers. Federal agencies are mandated to lease federal sites, accelerate clean energy deployment, prioritize AI infrastructure permits, develop transmission lines, interconnect AI infrastructure to the electric grid, ensure low electricity prices for consumers, and further allies and partners' AI infrastructure development. Private developers are required to fully fund construction, operation, and maintenance of the AI infrastructure, and uphold strong labor standards and procure domestically manufactured semiconductors used to power AI.
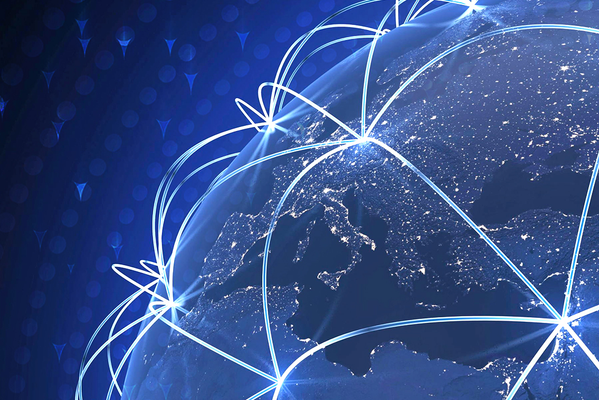
The AI Tracker is a tool that follows AI legislation, regulation, guidance, and more around the world in real-time. They have also created an eBook that highlights the most significant developments in AI regulation and legislation as we move into 2025 in the APAC, EMEA, and AMER regions. The eBook can be downloaded from the provided link.